PATH-DT-MSU Dataset
Description
Real biopsy and surgical material from various parts of the human digestive tract was used for paraffin blocks preparation. Microscopic examination was performed using microscope Leica DM2500 (Leica Microsystems, Germany). Microscope Leica DM4000B/DFC495 and scanner Leica SCN400 (Leica Microsystems, Germany) were used for high resolution histological images acquisition.
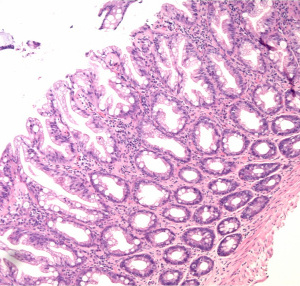 |
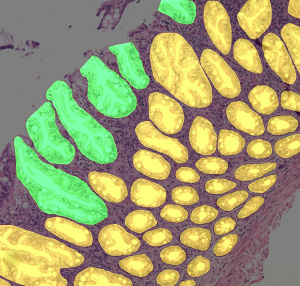 |
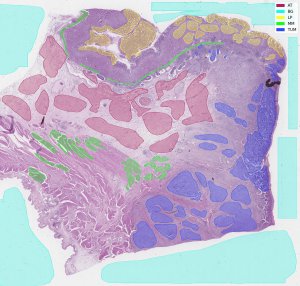 |
sample image from S1 set |
visualization of corresponding gland and "open" gland annotations from S1 set |
preview of whole slide image from WSS2 with visualization of annotation |
PATH-DT-MSU dataset was created to unite high-quality histological images of different parts of human gastrointestinal tract. It consists of several sets, containing different image types and annotations for different target tasks:
- S1: histological images of colon with annotations for segmentation;
- S2: histological images of stomach with annotations for segmentation;
- S3: histological images of esophagus with annotations for segmentation (planned);
- WSS1: whole slide images of colon polyps with annotations for segmentation;
- WSS2: whole slide images of gastric cancer with annotation for segmentation;
- WSR1: whole slide images with annotations for registration (planned);
All images are annotated, the annotation type for different sets is different according to the image type and target task. All images in sets for convenience are already split into train and test samples.
Versioning system and organization
PATH-DT-MSU is actively updated and expanded so for convenient usage each set supports versioning system. Every new version of set besides offering new images will contain all images from the previous version. Image annotations (including the number of supported classes of histological structures) can be modified and expanded from version to version. All outdated versions as well as the latest one are available for download.
The dataset is organized in sets and samples (train/test). Every source image is accompanied with annotations. We also include short medical description of each image.
Summary
set |
ver. |
tissue |
stain |
image type |
task |
number of images [train/test] |
magn. |
image size |
annotation |
description |
release date |
download |
S1 |
v1 |
colon |
H&E |
conventional [.png] |
segmentation (2 classes) |
20 [10/10] |
x10 |
2176×1632 |
pixel masks |
link |
23.12.2019 |
images + masks + previews (177 MB) |
v2 |
colon |
H&E |
conventional [.png] |
segmentation (2 classes) |
80 [40/40] |
x10 |
3263×2442, 2176×1632 |
pixel masks |
link |
01.03.2020 |
images + masks + previews (985 MB) |
S2 |
v1 |
stomach |
H&E |
conventional [.png] |
segmentation (3 classes) |
20 [10/10] |
x20, x5, x10 |
3263×2442 |
pixel masks |
link |
10.12.2022 |
images + masks + previews (720 MB) |
v2 |
stomach |
H&E |
conventional [.png] |
segmentation (3 classes) |
40 [20/20] |
x20, x5, x10 |
3263×2442, 1200×600 |
pixel masks |
link |
28.12.2023 |
images + masks + annotations (776 MB) |
WSS1 |
v1 |
colon |
H&E |
WSI [.svs] |
segmentation (5 classes) |
10 [5/5] |
x40 |
up to 59k×43k |
polygons |
link |
23.12.2019 |
images (2 GB), annotations, previews |
v2 |
colon |
H&E |
WSI [.svs] |
segmentation (5 classes) |
10 [5/5] |
x40 |
up to 59k×43k |
polygons |
link |
28.12.2023 |
images (2 GB), annotations, previews |
WSS2 |
v1 | stomach |
H&E |
WSI [.svs] |
segmentation (5 classes) |
10 [5/5] |
x40 |
up to 11k×90k |
polygons |
link |
23.12.2019 |
images (21 GB), annotations, previews |
v2 |
stomach |
H&E |
WSI [.svs] |
segmentation (5 classes) |
10 [5/5] |
x40 |
up to 11k×90k |
polygons |
link |
28.12.2023 |
images (21 GB), annotations, previews |
Download the Dataset
Please download the required sets and versions of PATH-DT-MSU using the links from Summary section.
Data Usage Agreement
You are free to use the provided data in your own research work. If you intend to publish research work that uses this dataset, you have to cite the references whenever appropriate.
Contact
For questions on PATH-DT-MSU dataset please contact Alexander Khvostikov: khvostikov@cs.msu.ru.
Our team
PATH-DT-MSU dataset was collected, prepared and annotated by Laboratory of Mathematical Methods of Image Processing, Faculty of Computational Mathematics and Cybernetics, Lomonosov Moscow State University and Department of Pathology, Medical Research and Educational Center (University Clinic), Lomonosov Moscow State University:
Alexander Khvostikov
khvostikov@cs.msu.ru
ORCID: 0000-0002-4217-7141
PhD, Researcher, Laboratory of Mathematical Methods of Image Processing, Faculty of Computational Mathematics and Cybernetics, Lomonosov Moscow State University
Andrey Krylov
kryl@cs.msu.ru
ORCID: 0000-0001-9910-4501
Professor, Head of the Laboratory of Mathematical Methods of Image Processing, Faculty of Computational Mathematics and Cybernetics, Lomonosov Moscow State University
Ilya Mikhailov
imihailov@mc.msu.ru
ORCID: 0000-0001-8020-369X
PhD, Trainee researcher, Department of Pathology, Medical Research and Educational Center, Lomonosov Moscow State University.
Nina Oleynikova
noleynikova@mc.msu.ru
ORCID: 0000-0001-8564-8874
MD, PhD, Researcher scientist, Department of Pathology, Medical Research and Educational Center, Lomonosov Moscow State University.
Olga Kharlova
olga.arsenteva@gmail.com
ORCID: 0000-0002-5909-1248
MD, PhD
Natalia Danilova
ndanilova@mc.msu.ru
ORCID: 0000-0001-7848-6707
MD, PhD, Senior researcher scientist, Department of Pathology, Medical Research and Educational Center, Lomonosov Moscow State University.
Pavel Malkov
pmalkov@mc.msu.ru
ORCID: 0000-0001-5074-3513
MD, ScD, Head of Department of Pathology, Medical Research and Educational Center, Lomonosov Moscow State University.
Acknowledgements
The work on PATH-DT-MSU S1 subset and preliminary versions of WSS1 and WSS2 subsets was supported by RFBR, CNPq and MOST according to the research project 19-57-80014 (BRICS2019-394).
Since 2022 the work on PATH-DT-MSU is supported by Russian Science Foundation grant 22-41-02002 “Value-added Analysis of Histological Images using Artificial Intelligence”.
Bibliography
2023
-
Alexander Khvostikov, Andrey Krylov, Ilya Mikhailov, and Pavel Malkov. Visualization and analysis of whole slide histological images. Lecture Notes in Computer Science, 13644:403–413, 2023. DOI
-
V. E. Karnaukhov, A. V. Khvostikov, and A. S. Krylov. Generative augmentation methods for histological image analysis in limited data conditions. Computational Mathematics and Modeling, 33(3):365–374, 2023. DOI
-
M. A. Penkin, A. V. Khvostikov, and A. S. Krylov. Automated method for optimum scale search when using trained models for histological image analysis. Programming and Computer Software, 49(3):172–177, 2023. DOI
-
N. D. Lokshin, A. V. Khvostikov, and A. S. Krylov. Augmenting the training set of histological images with adversarial examples. Programming and Computer Software, 49(3):187–191, 2023. DOI
-
A. S. Veshkin and A. V. Khvostikov. Multiscale content-based image retrieval for whole-slide histological images. Computational Mathematics and Modeling, 33(2):244–254, 2023. DOI
-
Sun Zhongao, Alexander Khvostikov, Andrey Krylov, and Nikolai Krainiukov. Super-resolution for whole slide histological images. In Proceedings of the 33nd International Conference on Computer Graphics and Vision, pages 609–619, Moscow, 2023. DOI
-
Nikita Yakovlev, Alexander Khvostikov, and Andrey Krylov. Method for automatic initialization of trainable active contours for instance segmentation in histological images. In Proceedings of the 33nd International Conference on Computer Graphics and Vision, pages 598–608, Moscow, 2023. DOI
2022
-
I. A. Mikhailov, A. V. Khvostikov, and A. S. Krylov. Methodical approaches to annotation and labeling of histological images in order to automatically detect the layers of the stomach wall and the depth of invasion of gastric cancer. Pathology Archive, 84(6):67–73, 2022. DOI
-
A. V. Khvostikov, A. S. Krylov, I. A. Mikhailov, and P. G. Malkov. Visualization of whole slide histological images with automatic tissue type recognition. Pattern Recognition and Image Analysis: Advances in Mathematical Theory and Applications, 32(3):483–488, 2022. DOI
-
O. L. Pochernina, A. V. Khvostikov, and A. S. Krylov. Semi-automatic algorithm for lumen segmentation in histological images. In Proceedings of the 32nd International Conference on Computer Graphics and Vision, pages 648–656, Moscow, 2022. DOI
2021
-
Alexander Khvostikov, Andrey Krylov, Ilya Mikhailov, Pavel Malkov, and Natalya Danilova. Tissue type recognition in whole slide histological images. CEUR Workshop Proceedings, 3027:50, 2021. DOI
-
I. Mikhailov, A. Khvostikov, Andrey S. Krylov, P. Malkov, N. Danilova, and N. Oleynikova. Development of CNN-based algorithm for automatic recognition of the layers of the wall of the stomach and colon. Virchows Archiv, 479(Suppl 1):OFP–15–004, 2021. DOI
2020
-
I. Mikhailov, A. Khvostikov, Andrey S. Krylov, P. Malkov, N. Oleynikova, O. Kharlova, and N. Danilova. Development of semi-automatic interactive algorithm for annotating histological images of colon epithelial neoplasms. Virchows Archiv, 477(S1):OFP–10–002, 2020. DOI
-
Alexander Khvostikov, Andrey Krylov, Ilya Mikhailov, and Pavel Malkov. CNN assisted hybrid algorithm for medical images segmentation. In ICBIP '20: Proceedings of the 2020 5th International Conference on Biomedical Signal and Image Processing, pages 14–19, New York, N.Y., United States, 2020. ACM. DOI
2019
-
A. V. Khvostikov, A. S. Krylov, I. A. Mikhailov, and P. G. Malkov. Trainable active contour model for histological image segmentation. Scientific Visualization, 11(3):64–75, 2019. DOI
-
A. V. Khvostikov, A. S. Krylov, I. A. Mikhailov, O. A. Kharlova, N. A. Oleynikova, and P. G. Malkov. Automatic mucous glands segmentation in histological images. ISPRS Journal of Photogrammetry and Remote Sensing, 42(2/W12):103–109, 2019. DOI
-
N. Oleynikova, A. Khvostikov, A. S. Krylov, I. Mikhailov, O. Kharlova, N. Danilova, P. Malkov, N. Ageykina, and E. Fedorov. Automatic glands segmentation in histological images obtained by endoscopic biopsy from various parts of the colon. Endoscopy, 51(4):S6–S7, 2019. DOI
|
|
|