Description
The material used was collected from 30 ore deposits of the CIS. Prepared samples of polished sections were analyzed using a Carl Zeiss AxioScope 40 microscope, photographing was carried out using a Canon Powershot G10. Samples represent the main ore associations and are categorized by deposit genesis.
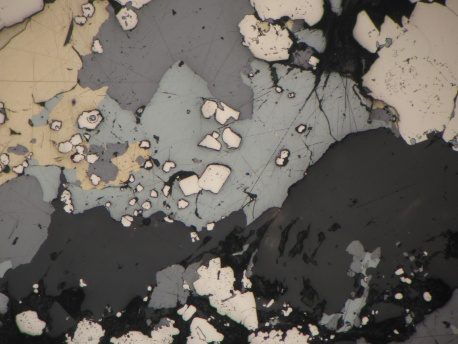 | 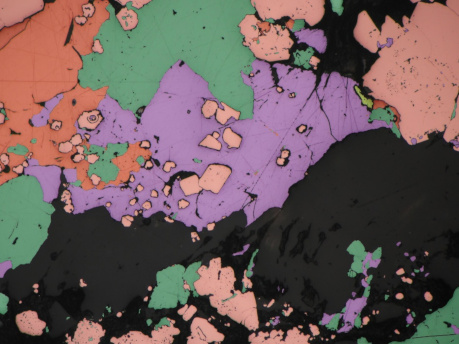 |
sample source image | corresponding overlay of source image and colorized mask |
LumenStone dataset was created to unite high-quality geological images of different mineral associations and provide pixel-level semantic segmentation masks. It consists of several sets:
- S1: association of hydrothermal ore of Berezovskoe deposit consisting of sphalerite, pyrite, galena, bornite, tennantite-tetrahedrite group, chalcopyrite minerals,
- S2: association of Layered Ultramafic Deposits (Deposits of the Norilsk Group), consisting of pyrrhotite, chalcopyrite, pentlandite, magnetite minerals,
- S3: common association of high-temperature hydrothermal ores consisting of pyrite, arsenopyrite, covelline, bornite, chalcopyrite, magnetite (ordinary and copper-bearing magnetite), hematite (secondary and hydrothermal) minerals (coming soon).
Versioning system
LumenStone dataset is actively updated and expanded so for convenient usage each set supports versioning system. Every new version of set besides offering new images will contain all images from the previous version. Image annotations (including the number of supported classes of histological structures) can be modified and expanded from version to version. All outdated versions as well as the latest ones are available for download.
Summary
set | version | number of images [train/test] | number of classes | magnitude | image sizes in pixels | release date | download |
S1 | v1 | 59 / 16 | 7 | x100 | 3396x2547 | 24.02.2021 | link1, link2 |
S2 | v1 | 23 / 6 | 5 | x100 | 3396x2547 | 24.02.2021 | link1, link2 |
Directory structure
The LumenStone dataset is split into sets and samples (train/test). Each set represents a stable mineral association that corresponds to the main types of deposits.
Every image in the dataset has 4 representations:
- source image,
- pixel-level ground-truth semantic mask (values 0, 1, 2… in the mask correspond to classes 0, 1, 2, …),
- colorized mask for simple visualization,
- an overlay of source image and colorized mask,
which are stored in folders imgs, masks, masks_human, masks_overlay correspondingly.
The short geological description of sets, as well as the description of all annotated classes of minerals and their color bindings can be found in
description.xls table. The current version of
description.xls can be found
here or
here.
The directory structure of LumenStone dataset is the following:
LumenStone
|- description.xls
|- S1
| |- v1
| | |- test
| | | |- imgs
| | | | |- 01.jpg
| | | | |- 02.jpg
| | | | | . . .
| | | |- masks
| | | | |- 01.png
| | | | |- 02.png
| | | | | . . .
| | | |- masks_human
| | | | |- 01.png
| | | | |- 02.png
| | | | | . . .
| | | |- masks_overlay
| | | | |- 01.jpg
| | | | |- 02.jpg
| | | | | . . .
| | |- train
| | | |- imgs
| | | | |- 01.jpg
| | | | |- 02.jpg
| | | | | . . .
| | | |- masks
| | | | |- 01.png
| | | | |- 02.png
| | | | | . . .
| | | |- masks_human
| | | | |- 01.png
| | | | |- 02.png
| | | | | . . .
| | | |- masks_overlay
| | | | |- 01.jpg
| | | | |- 02.jpg
| | | | | . . .
| |- v2
| | |- test
| | | |- imgs
| | | | . . .
| | | |- masks
| | | | . . .
| | | |- masks_human
| | | | . . .
| | | |- masks_overlay
| | | | . . .
| | |- train
| | | | . . .
|- S2
| |- v1
| | |- test
| | | | . . .
| | |- train
| | | | . . .
| | . . .
| . . .
Download the Dataset
Please download the required sets and versions of LumenStone dataset using the links from Summary section.
The all-in-one version of LumenStone dataset including all sets and versions is available for download
here or
here.
Data Usage Agreement
You are free to use the provided data in your own research work. If you intend to publish research work that uses this dataset, you have to cite the references whenever appropriate.
Contact
For questions on LumenStone dataset please contact Alexander Khvostikov: khvostikov@cs.msu.ru.
Our team
LumenStone dataset was collected, prepared and annotated by Laboratory of Mathematical Methods of Image Processing, Faculty of Computational Mathematics and Cybernetics, Lomonosov Moscow State University and Department of Geochemistry and Economics of Mineral Resources, Faculty of Geology, Lomonosov Moscow State University:
Alexander Khvostikov
khvostikov@cs.msu.ru
ORCID: 0000-0002-4217-7141
Laboratory of Mathematical Methods of Image Processing, Faculty of Computational Mathematics and Cybernetics, Lomonosov Moscow State University
Dmitry Korshunov
Dmit0korsh@gmail.com
ORCID: 0000-0002-8500-7193
Department of Geochemistry and Economics of Mineral Resources, Faculty of Geology, Lomonosov Moscow State University
Andrey Krylov
kryl@cs.msu.ru
ORCID: 0000-0001-9910-4501
Professor, Head of the Laboratory of Mathematical Methods of Image Processing, Faculty of Computational Mathematics and Cybernetics, Lomonosov Moscow State University
Mikhail Boguslavsky
mikhail@geol.msu.ru
ORCID: 0000-0002-1582-8033
Department of Geochemistry and Economics of Mineral Resources, Faculty of Geology, Lomonosov Moscow State University
Acknowledgement
In 2019, the work on the LumenStone was carried out with financial support from the Innovation Promotion Foundation, project UMNIK 14582GU/2019.
In 2021, the work on the LumenStone was carried out with financial support from the school “Brain, Cognitive Systems, Artificial Intelligence”, Lomonosov Moscow State University.
Bibliography
2020
A. Kochkarev, A. Khvostikov, D. Korshunov, A. Krylov, M. Boguslavskiy. “Data balancing method for training segmentation neural networks” // In: CEUR Workshop Proceedings, Vol. 2744. 2020, pp. 1−10.
Д. М. Коршунов, А. В. Хвостиков, А. В. Кочкарёв, М. А. Богуславский, А. С. Крылов. «Использование алгоритмов глубокого обучения для сегментации и анализа минералов на изображениях аншлифов» // в: Новое в познании процессов рудообразования: Труды молодых учёных, посвящённые 90-летию ИГЕМ РАН. 2020, с. 66−68.